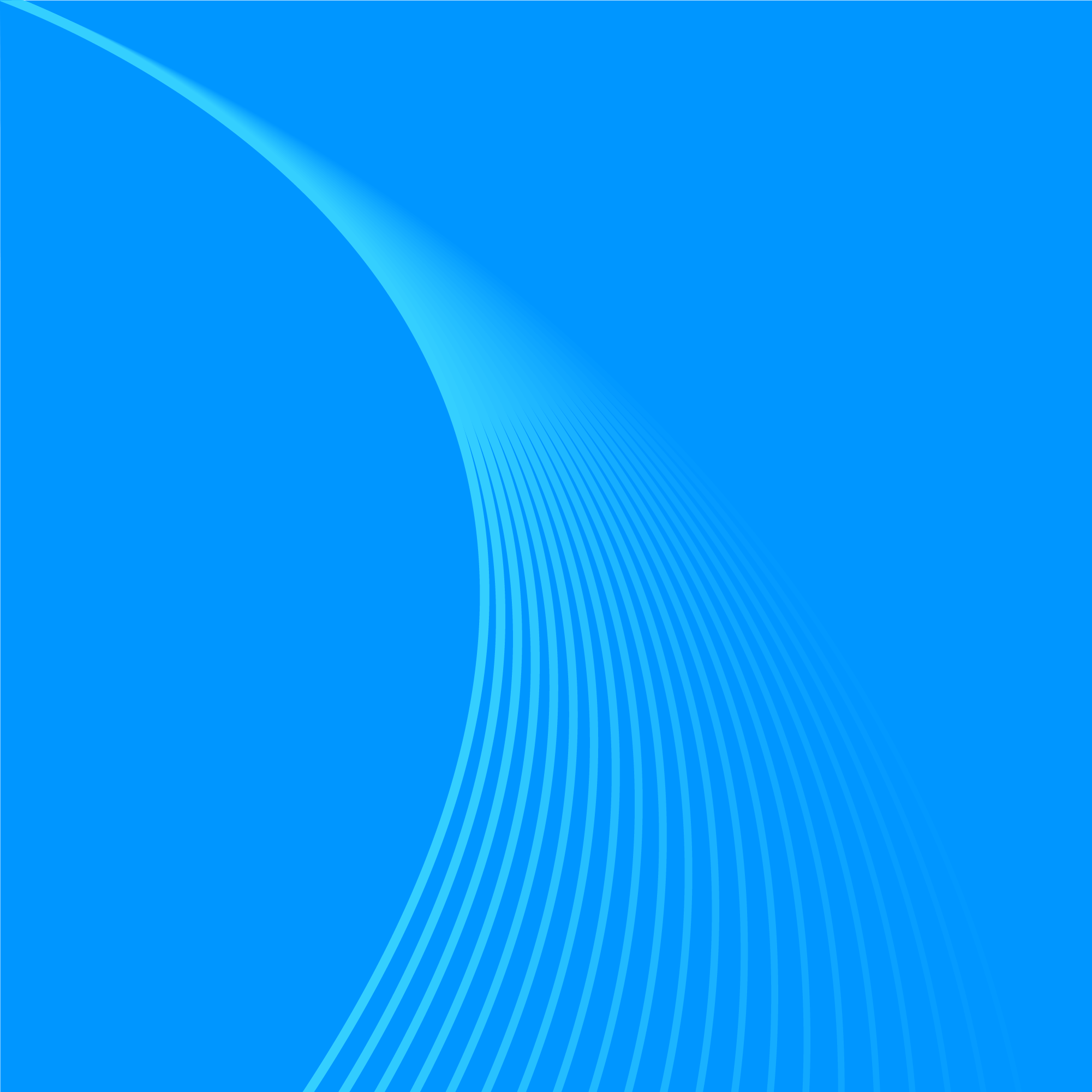
Originally published in Clinical Leader
AI has gone from an underused tool in clinical trials to one with real and valuable applications. But the transition from concepts to standards is far from complete. There’s potential for life sciences companies to do much more with AI over the next several years — representing an exciting prospect as well as a strategic challenge.
Where are we now? In Q3 2022, Point B surveyed 100 leaders who are implementing AI in their organizations. The findings confirmed our outlook: Most life sciences organizations—even early adopters of AI — still need to make a significant transition to leverage AI advances. Specifically, executives overseeing clinical trials are looking to invest in a few key areas.
What’s driving investment in clinical trial AI?
Our study revealed that executives have three major AI drivers on the clinical side*:
- 61% Increasing clinical trial efficiency
- 57% Improving trial design and inclusion/exclusion criteria
- 64% Increasing trial participant retention
These are challenges where the current tools and technology, standards and regulations are developed enough, and mesh closely enough, to leverage AI. Executives overwhelmingly noted that reducing the time to file and gain approval was the primary key business outcome (86%) of investing in AI. When asked what types of AI or technologies are currently being used, biometrics, machine learning, and expert systems were top of mind, but overall clinical trial functional areas are behind in investment compared to other functional areas. However, the data shows that there are aggressive plans to invest in the next 3 – 5 years.
For example, companies are using AI to accelerate patient identification and recruitment—going through health records to identify biomarker data relevant to a particular trial. AI is helping pharma and biotech companies identify the best sites for clinical trials based on past site performance and enroll patients accordingly. Big data techniques are being applied to pharmacovigilance adverse event detection. And AI is also in the early days of being applied to clinical study design. Based on performance data from past trial designs, AI can streamline trial design and protocol development.
What are executives’ biggest challenges?
Obstacles to broader use of AI show up in what leaders see as significant limitations*:
- 48% Technology Maturity
- 40% Increasing Clinical Trial Efficiency
- 37% Internal Talent
*Percentages exceed 100% because of multiple responses
Diving deeper into these findings, we see a few contributing factors:
Mature AI tools and technology from retail, social media and other industries aren’t transferrable for clinical use.
Most clinical data is not yet easily accessible for AI. Studies show that between 40 – 70% of the work in data science is in accessing the data, understanding it, and cleaning it up. High-quality data is essential to using AI in such processes as evaluating the impact of inclusion and exclusion criteria on patient demographics, predicting the value of typical endpoints and identifying new ones, and exploring scenarios for complex adaptive trials.
The sexy part of AI—running a set of algorithms and coming up with actionable results—is the “easy” part. The tougher, more time-consuming work is in preparing high-quality, underlying data to run those algorithms against. And while companies are investing in digitalization now, everything up to the point of digitalization is legacy data buried in PDFs, Word documents and image files. From an API standpoint, most of the information needed to process and integrate data is locked up in historical documents.
To complicate matters, data structures and standards are highly variable across organizations—and even within organizations. Simultaneously, more data is being demanded by the FDA and EU regulatory bodies, which recognize past protocol documents as not being detailed enough to define a reproducible clinical trial. These regulatory bodies are beginning to require greater detail and, often, multiple amendments that can easily run 100 pages.
Defining common terminology and data structures can be a heavy lift, but your organization doesn’t have to go it alone. Significant progress by third-party standards organizations (CDISC, Transcelerate and Accumulus are just a few of many) will make it possible to adopt one of their versions instead of investing the time and money to do the work in-house.
Commercial tool vendors are beginning to build AI products that can be leveraged for some aspects of clinical trials, but most are essentially a combination of the same things standards organizations and life sciences companies are doing. Progress toward more mature tools has been slowed by questions of standardization and terminology. Case in point: In order to make a valuable AI tool that will fully support protocol design, it must reflect the structure of the protocol—including a controlled vocabulary. This capability has been beyond the purview of many tool vendors to date.
How to Move Forward?
The ability to develop and use AI in more complex clinical applications depends on three factors: new technology, industry-wide standards and evolving regulations. These three factors are progressing at different paces, making it challenging for life sciences companies to plan for the future.
Considering its current state and future potential, how does AI impact your day-to-day decisions? How will you transition from where you are to where you want to be as AI tools, standards and regulations mature over the next few years?
As you plan for the future of AI in your organization, it pays to keep several key considerations in mind:
Build-versus-buy has an explicit time dimension.
In the early days, life sciences companies had no practical options to buy AI tools. To create them, they had to hire their own data science teams, set up internal development infrastructures, and essentially do the data conversion, exploratory analysis and hypothesis testing themselves, a time consuming and costly process.
Life sciences companies are in the process of shifting their AI investments from the early days of “100% build” to a future that is closer to “100% buy.” In a well-established commercial tool environment, doing it all yourself would be like building your own ERP system — something nobody does. A “buy” approach can increase implementation speed and functionality while decreasing maintenance and acquisition costs.
Where are you today?
How you move forward will depend on where you begin. Early adopters have legacy tools and technology to deal with. Partnering to make the most of existing AI investments and determine when and where to shift from “build” to “buy” as tool vendors and standards organizations mature is critical.
If you have built AI, the questions are: What happens in the transition? How do you make a smooth, strategic shift that’s right for your company as AI tools, standards and regulations evolve?
For those just entering the AI world, you’ll want to look both inside your organization and at the vendor landscape to identify the best solutions given your risk profile. What’s your best use of AI? What skill sets and tools should be developed in-house? What should you buy?
If you are starting your clinical trial AI journey today, the questions are: Who are my best partners? How do I know they’ll address my needs? What, if anything, needs to be developed in-house?
What's next?
The market shake-out will continue as technologies emerge, fade or get acquired. We expect market turbulence to continue as AI technology and standards mature. Within the next several years, we expect availability of more advanced, core functions for clinical use, such as protocol design tools
The AI trifecta will become integrated and inseparable.
The trifecta of AI influences—tools/technology, standards and regulation will mesh in ways that make it easier to place your bets and make informed, forward-looking decisions.
The future of clinical trials is fully digital.
Advances in AI technology and standards will support increasingly sophisticated tools to accelerate drug development. The power of AI will enable companies to reach more participants and increasingly diverse populations, boost participant retention, produce faster trials at lower costs, and increase reusable data. It will play a lead role in increasing the speed of getting treatments to market, giving patients access to new drugs that could change, or even save, lives.
Today’s AI is on a journey toward a fully digitalized clinical trial future—a journey your company is likely to navigate over the next few years. Now’s the time to assess where you are on the journey as well as where you want to go. Develop strategies and internal strengths accordingly. Evaluate the vendors in this fast-changing landscape and find partners that will help move your clinical trials forward. Choose the right use cases. Apply the right technology. And stay the course. The journey will be worth it.
RELATED INDUSTRIES
RELATED SOLUTIONS