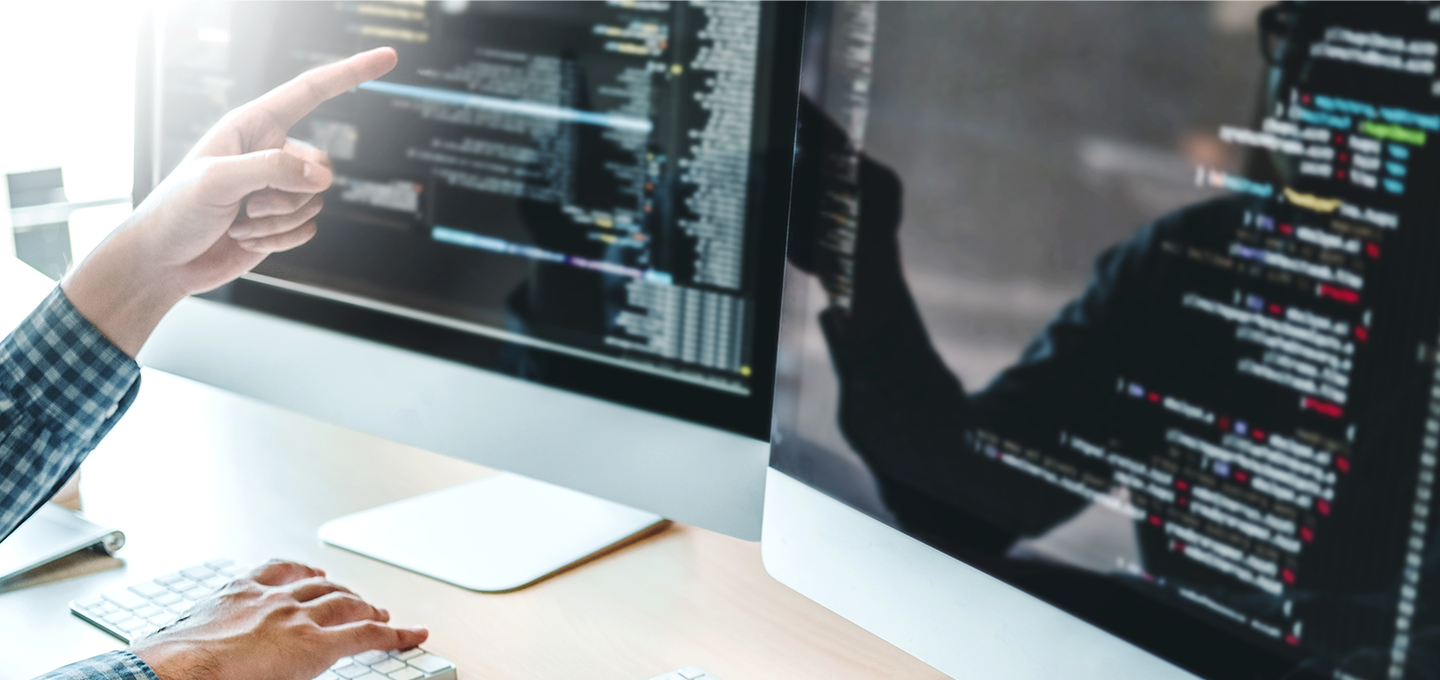
Governance is the one practice that can help you prevent data anarchy across your organization. It includes all actions taken to ensure data is accurate, secure, reliable, and available to the right people at the right times, making it a critical enabler of an effective data supply chain. Having a holistic and well-executed data governance capability drives business outcomes, mitigates risk, and eliminates chaos.
But establishing sustainable governance is much easier said than done. According to a recent GartnerĀ® report , āthrough 2025, 80% of organizations seeking to scale digital business will fail because they do not take a modern approach to data and analytics governance.ā 1
Symptoms of failing to take a modern approach to data and analytics governance include an inability to identify and achieve quantifiable business value, a lack of collaboration between business and IT, and failure to make a self-sustaining governance capability.
Clean and reliable data enables business leaders to make well-informed decisions. Whatās more, a careful data governance strategy is scalable ā which is essential for making the right decisions in a fast-growing business. By investing in a data governance approach that emphasizes flexibility and adaptability in response to changing needs and dynamics, youāll build a sustainable capability that can be applied to a variety of business challenges.
Through 2025, 80% of organizations seeking to scale digital business will fail because they do not take a modern approach to data and analytics governanceā
Avoiding the Pitfalls
One major roadblock to tackling data governance is often, ironically, failed past efforts to tackle data governance. Itās easy to spend a million dollars (or more!) on a high-tech tool or a specialized outside team and end up with hardly anything to show for it. Those all-too-common outcomes create an understandable aversion to investing in large-scale data governance projects. So itās essential to use proactive change management practices to earn trust and buy-in incrementally.
Incremental buy-in is also crucial for achieving the sweet spot that combines business value, speed, and adoption. Striking that balance is crucial, and this is where most organizations flounder.
The first step toward achieving balance and accountability is involving a multidisciplinary team from the start, rather than developing the model from one department. Because the technology involved in data governance is often complex and expensive, IT usually leads the project ā while business leaders often believe mistakenly that technology alone will create an efficient and scalable governance strategy.
Another common pitfall is the idea that an organization can develop a data-governance program in a vacuum and then impose it on the wider organization as a one-size-fits-all model. On the contrary ā stakeholders from different departments should drive the requirements from the start. And the right program will be flexible enough to meet varied needs across the organization.
7 Steps to a Successful Data Governance Model
1. Assemble a core team.
Establish a working group of key stakeholders from across the organization. That should include business leaders, IT, production/manufacturing, and leaders from every department that will make decisions based on data and insights.Ā
2. Know your objectives.
Clearly define the specific business outcomes the data governance program is meant to achieve. These should align with the analytics product strategy and directly support the data supply chain required to produce those products.
3. Assess your resources and set priorities.
Determine your organizationās drivers, opportunities, capabilities, and challenges. Establish a backlog of projects and prioritize them based on feasibility, effort and business benefit.
4. Call in reinforcments
Take stock of knowledge gaps and identify what you may need guidance to achieve. Find the right partner(s) to help identify key data sets, define DG processes and technology needed.
5. Finalize your lineup.
Select an initial organizational structure with clearly defined roles and responsibilities for each member of the DG team. Establish the scope for each person, business function and process. Appoint a DG Program Manager to oversee the entire process, who subsequently works with sponsors from each function to understand specific needs and capabilities.Ā
6. Set your sights and begin.
Select the initial project from the backlog, define success metrics, create a work plan and launch agile execution.Ā
7. Share the good news.
Early and often! share the model and goals widely throughout this process. Communicate the modelās value broadly to the entire organization to garner buy-in and encourage ongoing feedback and improvement to the model.Ā
Real World Impact: Establishing a Large-Scale Governance Framework
The data and analytics experts at Point B help clients build data governance structures that will last and sustain their value.
One such client, a global aerospace and defense technology company, faced an increasingly complex and siloed data ecosystem after a series of mergers and acquisitions that had resulted in a patchwork of manual processes and tribalization. This not only impeded business tooling, data, and process harmonization, but also complicated the generation of actionable business insights.
One of the key deliverables for this client was a data governance framework. To build that framework, the Point B team:
- Established an initial core working team to help define the Data Governance Future State.
- Identified the key pain points and considered the enterprise goal of a unified data environment that would allow for a measure of data autonomy across business segments.
- Determined the type of data governance model that would meet the needs of the business based upon various inputs and analysis. In this case, the best fit was a blended central/federated model.
- Defined three primary groups of stakeholders with specific roles and responsibilities, as well as the scope for each group.
- Crafted the Data Governance Playbook that informed the Data Governance Organization how to identify and execute against specific Data Governance Use Cases.
The result was a data governance framework, free of silos and unnecessary processes, built to scale and provide more value as the organization continues to grow.
RELATED SOLUTIONS