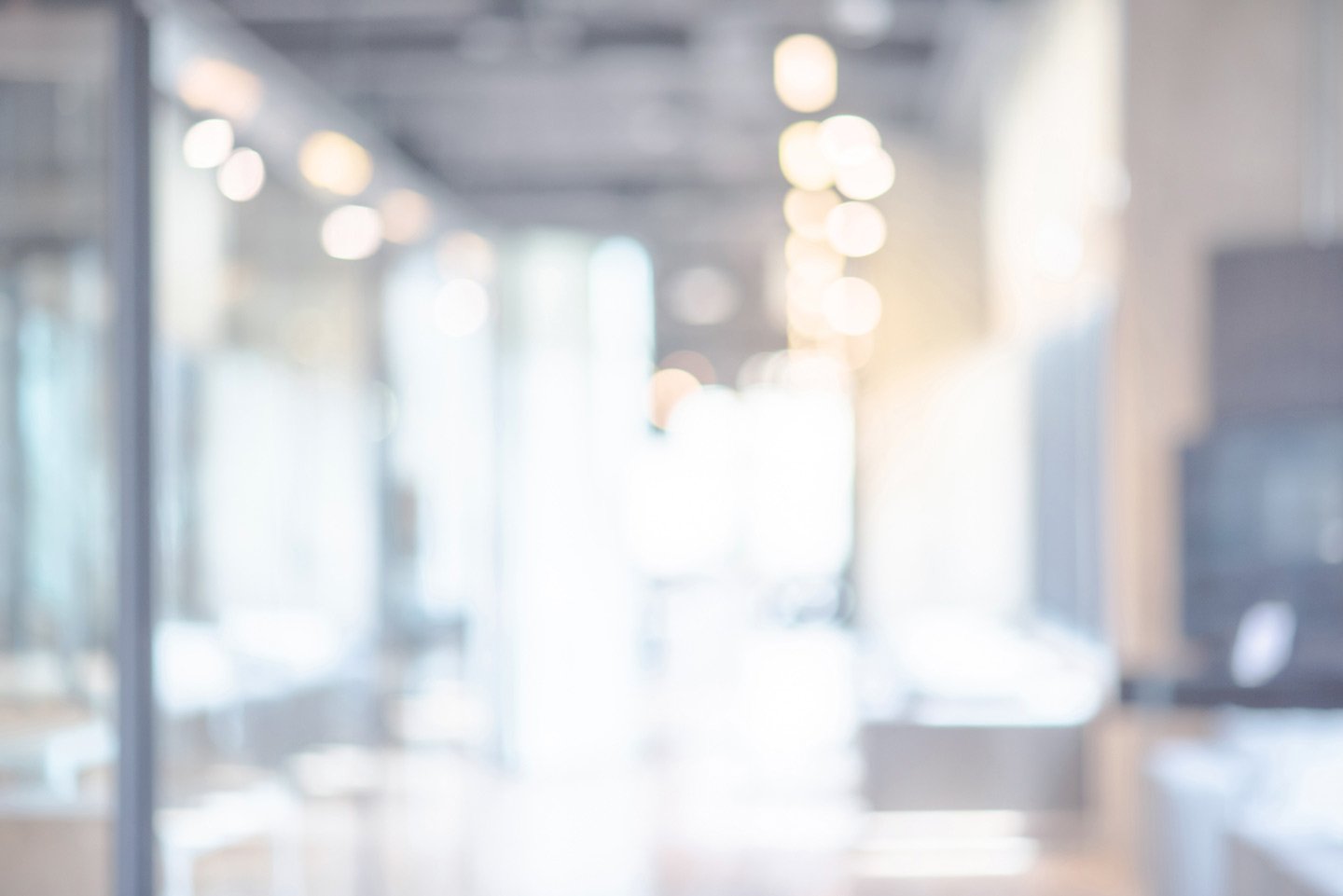
Wondering about the current and future state of AI in life sciences? You’re not alone. Now more than ever, we’re hearing from customers who are curious about how AI can help them develop therapeutics and optimize business processes. Point B set out to understand the current and future state of AI in life sciences organizations by surveying executives across the emerging biotech, pharmaceutical manufacturing and medical device and technology industries. Here’s what we found: It’s not a question of if but how.
Every organization surveyed is either currently using or planning to use artificial intelligence, machine learning, or some form of automation to manage and interpret their vast quantities of data, with an eye toward accelerating clinical trials and business operations. Yet fewer than half (43%) consider their organizations prepared for successful deployment of these technologies.
Why invest?
It’s about speed – and time is money. A therapy's profitability and ROI is dependent on how quickly it can get to market. Executives believe AI can accelerate the product development life cycle, all the way through to submission.
But efficiency is just one of many factors that impact speed to market. It’s also critical to mitigate the bottlenecks that most often lead to delays, including site selection, trial recruitment, deviations, emerging diversity regulatory requirements, & more.
When asked which applications or capabilities AI is delivering today, less than half of executives mentioned predictive analytics. While this may seem surprising, it maps to what we are hearing from customers. Most organizations are still early in their maturity when it comes to using AI and advanced technologies. The leading capability AI currently provides is data gathering and automation, an integral first step, since advanced AI isn’t possible without the right data structure and protocols in place.
Digitization of information is just the beginning. We’re helping organizations or functional groups understand AI’s full potential and how they can reach the next step in their journey. The ultimate goal is to unlock historic analytics, helping organizations mitigate risks and manage all variables. Or to help customers bring new, digital data flows into their management and governance structure. When all information is available and organized in a digital format, organizations can move on to more complex applications like predicative analytics.
Most organizations are still early in their maturity when it comes to using AI and advanced technologies.Â
Point B’s Data and Digital Protocol Maturity Model
Our maturity model helps us understand where customers sit today and where they want to be tomorrow.
An Eye on Clinical Trials
Currently, executives are focused on efficiency as the path to improving speed to market. This is particularly true in the clinical trial setting. Executives are applying AI and advanced technologies to reduce or remove common regulatory submission and approval roadblocks.
Key Challenges
Of those who have implemented AI or AI technologies, only 44% experienced moderate to severe challenges, with 15% citing that they experienced no challenges at all.
Key challenges will shift with technology, process, and the regulatory environment. Today, process maturity was cited as a significant problem for both AI users and non-users (40% and 50% respectively). This translates to data digitization challenges and the up- and downstream processes needed to feed information into advanced technologies. Diagnostic errors will naturally decrease as technology maturity and threshold standards evolve. Read more about the essential, but unsexy, side of AI here.
The Talent Challenge
Hiring or developing the right internal talent is a top challenge. Whether your strategy is build, buy, or a combination, you will need specialized talent for ongoing program support. The specific team composition and talent level will vary based on your approach, but
you will need some level of internal data science talent to ensure the success of your efforts. This presents a big barrier and challenge due to the low supply of data scientists compared to market demand.
- Building? Data scientists are required if you plan to build your own advanced technology and analytics capabilities.
- Buying? A seasoned and sophisticated business analyst with a mix of data and advanced analytics expertise can help guide you through use cases, vendor selection, content digitization, and other key needs.
While the talent market is tight right now, the future looks bright. The U.S. Bureau of Labor Statistics sees strong growth in the data science field and predicts the number of jobs will increase by about 28% through 2026. That will mean roughly 11.5 million new jobs in the field.
When asked about the status of an internal team to support AI-readiness, all executives reported that they either have one or are considering it.
Talent to Management a Portfolio Approach
Many use cases require multiple technologies to fully meet requirements. This presents a problem as data science skill sets are getting more specialized, making building and maintaining a team that can cover the full range of functionality increasingly difficult. Vendors face the same problem, which is leading to increased specialization and best of breed solutions.
We expect this picture to look very different in just a few months. Since it’s impossible to stay in front of rapidly proliferating technologies, it’s most critical to focus on continued education around your business case and buy-vs-build approach. When it comes to perfecting the use of technology, apply the 80-20 rule and consider how you can strategically separate hype from reality.
For example, at the time of this survey, ChatGPT had yet to burst onto the scene making it impossible to account for how NLP would change our results today. And we continue to see the technologies’ boundaries pushed.
While it is easier to wait until technology is adopted and integrated into the commercial vendor landscape or enterprise suites, it comes at the cost of delayed implementation and progress. However, that time can be used wisely to build a strong foundation with structured content management (SCM), small scale experiments, internal talent development, or recruitment.
Conclusion
We’re no longer wondering if you should invest in AI but how. Executives are already investing, despite challenges setting up the right internal infrastructure to support their investment.
Technology is constantly evolving, and life sciences organizations will need to evolve with it.
RELATED INDUSTRIES
RELATED SOLUTIONS