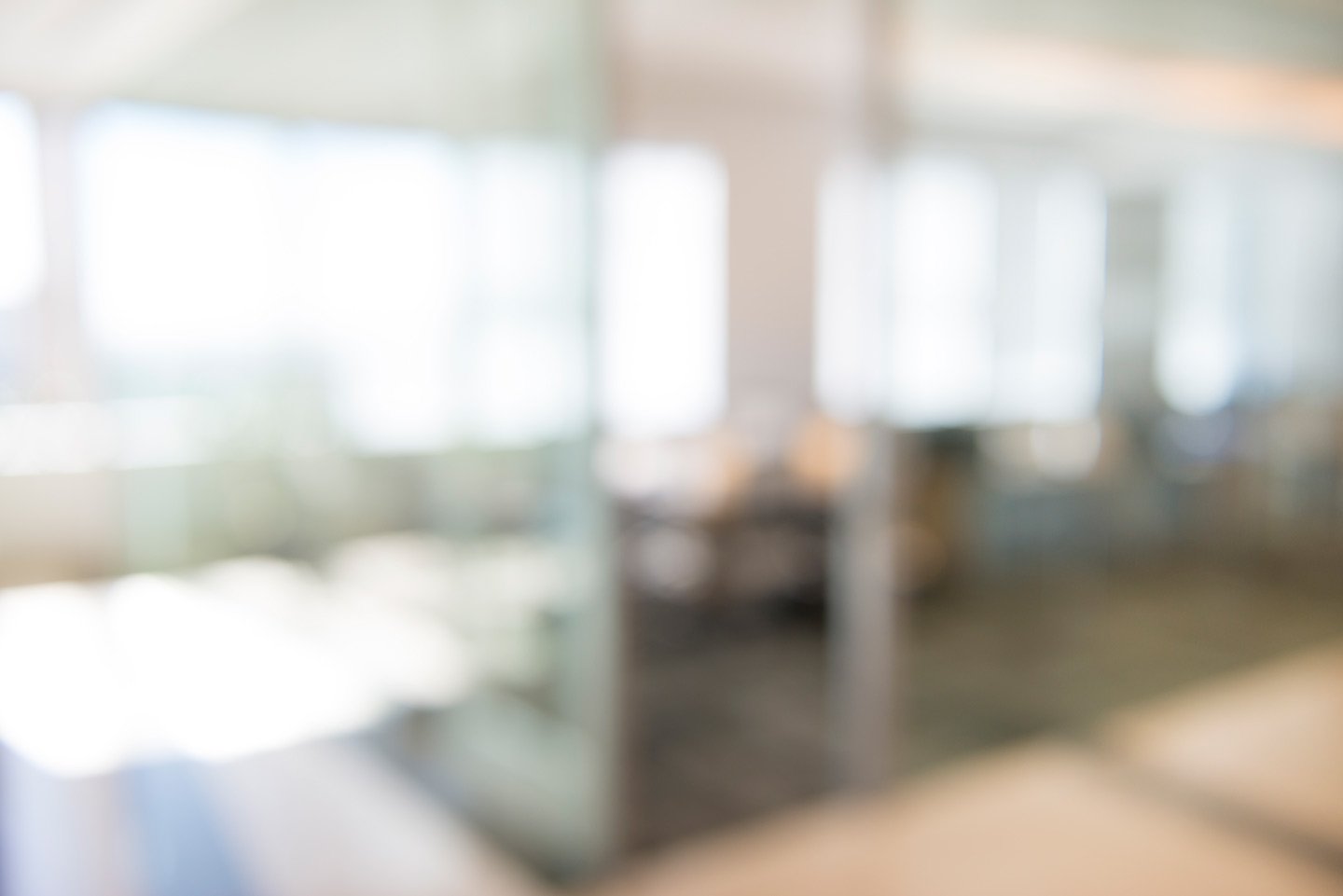
Key Takeaways
- Artificial Intelligence is only as strong as your up and downstream data and processes
- New ways of working and managing data should be established with AI in mind – and before AI implementation
- Organizational data consensus and culture is a critical step
- To create buy-in or avoid feeling overwhelmed, get started with a time-boxed proof of concept
Over the next decade, we’ll see artificial intelligence and other innovative technologies advance and even transform life sciences, from discovery and clinical development to regulatory submissions to commercialization. While applications of AI are still being developed, there are valuable uses you can implement now and lessons to build on. But are you ready?
The vision and value of AI is expanding. Life sciences companies are on a steep adoption and learning curve. Early adopters are investing in AI to enhance speed-to-market and quality across the product lifecycle. It’s only a matter of time until companies apply AI at scale across entire product portfolios and value chains—discovering, developing and delivering therapeutic breakthroughs in ways that have yet to be imagined.
But you can’t reap AI’s transformative powers with today’s ways of working and managing data. The promise of AI depends on fundamentals that can’t be skipped or short-cut. It takes foundational work and investments in data model development, standards, governance and processes to deliver on AI’s potential.
Missing Fundamentals, Missed Opportunities
In the rush to embrace exciting technology, AI readiness fundamentals are often overlooked. Many companies come to an abrupt halt as they discover that foundational elements required for success are either incomplete or missing altogether.
AI readiness is critical to getting value out of this type of technology across the long, complex product development life cycles common to life sciences. For example, faster FDA filings and submissions cannot be accomplished in isolation from upstream data requirements like clinical trial protocols. We see customers pausing implementation, pivoting to invest in data governance, data dictionaries, metadata, custom templates and taxonomy to make their AI more successful.
Tacking AI technologies onto business-as-usual won’t work. Instead, you'll need to build a foundation that aligns data management, processes and organizational structures with AI technologies in order to maximize benefit from them.
So How Do You Begin?
First things first: practical steps to AI readiness
AI is not a sprint. It’s a journey that requires alignment across people, data, technology, information architecture, and business processes. We believe in building a foundation that supports early wins, creating momentum, business value and buy-in while providing a clear path to achieve longer-term AI goals. Here's how.
What Are Your Goals? And Your Gaps?
It may sound elementary, but it’s crucial to begin with a clear and shared understanding of what you want to achieve. Ask yourself:
- Where will AI deliver the greatest business value?
- Where are we struggling with manual, repetitive work?
- Where do we have large volumes of information that can be mined?
- How can we enable faster development, insight generation and execution of work?
- Where are we using outdated technology today?
Maybe it’s taking too much time and money to develop your protocol amendments, or you need to summarize and submit clinical study reports faster. Maybe you want to increase your speed in submitting full dossiers for new products to regulators or establish a better-defined source of truth. Since AI has the potential to address a variety of critical data issues across the product lifecycle, narrowing your focus will help you ensure the right solutions.
Next, assess where you are with data and content. Where are your governance and system processes today? How manual are they? What technology is involved? This gap analysis will uncover areas you can address for greater AI readiness.
Get Your Data Ready and Do It Right the First Time.
We can’t overstate it: Success in AI demands attention to the quality and integrity of the data flowing into your technology. If there’s one place where we see companies shortchange themselves, it’s here. Your AI performance will be as strong or as weak as the quality and management of your data.
It’s also critical to get your data right the first time. Going back to clean or reorganize it is extremely difficult but often needed for legacy products. Adopting standards like those from CDISC (Clinical Data Interchange Standards Consortium) can help. We encourage companies to invest in data standards and governance as early as possible in the clinical trial process because serious downstream barriers occur without the right processes in place.
Keep The Big Picture in Mind.
AI calls for seeing the big picture, even as you address a narrower slice of it. You need to look at the up and downstream impact across the product lifecycle. But just because pulling on one string impacts another part of the business doesn’t mean you should not invest in improvement: just be aware of the interdependencies. Thinking holistically but acting in a focused way is the best approach.
Create Language Consensus and Consistency.
AI is more than coding and programming. It requires consensus on taxonomy and terminology, which can vary widely from one product and stakeholder group to another. New standards, including defining metadata and data dictionaries are necessary. Getting alignment across functional areas on those standards is the most challenging part.
These are issues that put your people at the center of change. The implementation and application of AI technology is rooted in a very human element: language. The process requires multiple departments and stakeholders to agree on a set of standards. That may not be easy when, historically, there’s been little need for consistency from group to group. Companies often underestimate the amount of time it takes to align key stakeholders on language alone.
Change management, in addition to a set of taxonomy and terminology standards, is needed to create consensus.
Develop an integrated approach that builds ownership.
AI requires buy-in from an array of extremely specialized professionals across disciplines. Integrated planning and clear ownership create the level of accountability you need for AI to succeed. This takes strong sponsorship, particularly at the executive leadership level.
Here are a few activities you can do to build an integrated approach and ownership:
- Business problem and use case analysis
- Gap assessment and business case development
- Cross-functional initiative design and planning
- Business requirements development
- Data standards, governance and information architecture
- Technology strategy and vendor selection
- Implementation planning, execution & change management
We find that leaders are best able to see the need for executive focus and accountability when they look at AI through the lens of business objectives. It takes input and inclusion from the bottom-up, including two-way communications with diverse stakeholder groups. What’s in it for them?
Determining The Right Technology And Partners.
We recognize that AI is one of many advanced technologies. Machine learning, natural language processing (NPL), natural language generation (NLG), robotic process automation (RPA), structured content management, and auto-content generation are all valuable tools that can create speed and efficiency for enabling products’ go-to-market. Many of these require similar data standards, process definition and internal alignment. Determining which technology is best suited for the use cases in scope is a critical step in the journey – AI is not necessarily right for all situations.
The AI vendor landscape in life sciences is rapidly evolving. Vendors and products cover many categories, including process automation, structured content management, master data management and governance, and more. The right vendor will save you time, money and headaches while optimizing business value. We help life sciences companies navigate this complicated selection process and build strong partnerships with vendors that are best for them.
Where Do You Begin?
Build a time-boxed proof of concept.
When clients aren’t sure how to start - or if it’s even worth the investment - we suggest trying a time-boxed proof of concept. It’s an effective way to show how it could work and what’s achievable to a variety of stakeholders. We can quickly design and showcase new ways of working or applications of new technology. Tackling a specific problem on a very small scale lets you learn from that experience, then refine and scale with an iterative approach that will increase the success of any future plans.
It's “when,” not “if.” Grow your AI maturity level over time.
Every organization has its own AI needs and objectives. While it can be tempting to keep up with the trendiest technology, it’s far more important to understand what AI can do for you and your goals. You’ll also need the data readiness, infrastructure, governance and organizational strategy that will enable AI to meet your goals. AI is an evolution in maturity—understand where you are in the journey and take steps forward.
It’s still early days—with years of discovery and amazing opportunities ahead. What better time to master the fundamentals?
RELATED INDUSTRIES
RELATED SOLUTIONS